When calculating damages for future periods – such as for lost profits, loss of income in wrongful death cases, etc. – experts are often charged with expressing an opinion as to what would happen in the future but for the wrongful act. These projections typically rely upon either industry trends or on the historical operating results of the injured party. Four of the most commonly used projection conventions when relying upon the operating results of the injured party are: the mean, the median, exponential smoothing and regression analysis. This blog post will define each of these conventions and discuss the pros and cons of each.
The Mean
The mean describes the central tendency of a set of numbers and is calculated as the arithmetic average. When there is only a limited amount of historical data available and no clear trend of growth or decline exists, relying upon the mean may be the most reliable method to project future periods. However, if more historical data is available and/or there is a clear trend of growth or decline shown in the historical data of the injured party, then using the mean will render projections that are less reliable or indicative of the future than other projection conventions and will be difficult for the expert to defend.
The Median
The median also represents the central tendency of a set of numbers, but in this convention it is calculated by reference to the middle number after the set of numbers are arranged from highest to lowest in value. Because the inclusion of extreme outlying values (e.g., if the historical data is 5, 6, 8, 9, 40) can render distorted projections, relying upon the median may be the most reliable convention to project future periods. However, once again when there is a clear trend of growth or decline, using the median will produce projections that are less reliable than exponential smoothing or regression analysis.
Exponential Smoothing
The mean and the median conventions treat each observation equally. The exponential smoothing convention (also known as weighted averaging) assumes that the oldest observation should receive the least weight and the most recent observation should command the most weight in projecting future performance. It applies a weighting factor to each historical observation and then calculates an arithmetic average of the weighted historical observations. For example:
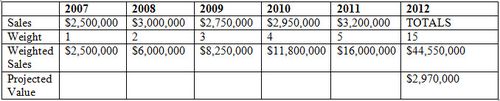
Use of exponential smoothing can be particularly effective if sufficient historical data exists and there are no extreme outlying values.
Regression Analysis
Regression analysis uses mathematical calculations to fit a line or curve to a set of historical numbers. The more common form of regression analysis used to project future periods is known as trend-based regression analysis or least squares analysis. This methodology seeks to fit a straight line to a set of historical numbers and then project future periods along that line. Like exponential smoothing, regression analysis can be particularly effective if sufficient historical data exists and if there are no extreme outlying values.
When calculating future damages based upon historical information, experts may rely upon a variety of conventions and projection methods. This post has discussed four of the most commonly used conventions – the mean, the median, exponential smoothing and regression analysis. In the next part, I’ll provide specific examples and show how each method impacts the damages calculation under each example.